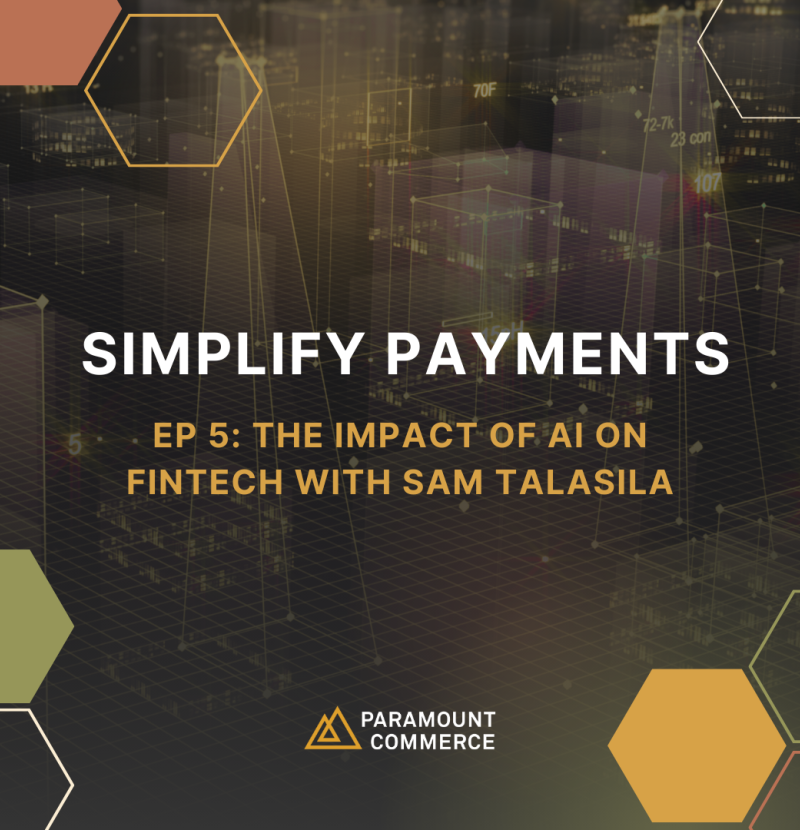
Simplify Payments Podcast - EP 5 (The Impact Of AI On Fintech with Sam Talasila)
In this episode, we dive into how artificial intelligence is transforming the fintech industry. We explore the benefits, challenges, and future implications of AI in fintech. And we’ll also explore how fintechs can leverage AI to enhance customer experiences, reduce fraud, and streamline operations.
Guest: Sam Talasila, Head of LLMs at Wealthsimple
The Simplify Payments Podcast, presented by Paramount Commerce, is a podcast series that takes a closer look at new and emerging financial technologies and practices with some amazing industry experts.
Please like, comment, and share this video. Also, stay up to date with our content by subscribing to our YouTube channel.
Full episode transcript:
Varad Mehta: Hello everyone, and welcome to the Simplify Payments podcast presented by Paramount Commerce. I’m your host, Varad Mehta. In Simplify Payments, we take a closer look at new and emerging financial technologies and practises with some amazing industry experts. In the fifth episode, we take a closer look at how artificial intelligence is impacting the fintech industry. Our guest for today’s episode is Sam Talasila, the Head of LLM’s at Wealthsimple. So please sit back and enjoy the show. So Sam, how we begin this podcast, as always, is by asking our guests a few fun questions. So I had a couple lined up for you. The first one is What does a ride on the Honda CBR 400 F4 feel like?
Sam Talasila: Awesome Varad. It’s nice to be here. It’s clear you’ve done your homework. So you’re pulling into my past where this brief period while I was at university, a few of my friends started getting into motorcycling. That was, at that time, one of the scariest things I’ve done. The first bike that I ever got after getting licenced was this pocket rocket 600 cc Honda race-oriented bike. The first time I rode it was in private where no one was around because I didn’t want them seeing me fall flat. But after that first week where I took it around being very gingerly, the idea that you have so much power and instantaneous control and access to that power just felt so free. Just felt so free. There’s nothing like having a nice spring, late spring day where it’s not too hot because you’re all geared up and off in the boonies somewhere and just you going down and experiencing curves. It’s just, yeah, that’s freedom, man. That’s freedom.
VM: I love it. What does the Honda feel like, a 400 F4? It feels like freedom is what Sam said. I love it. My last question would be, if you could apply your love for data science or data analysis to another industry, what would it be and why
ST: I used to do this in fantasy basketball
VM: Nice.
ST: That was fun, but not very rewarding or successful because with fantasy basketball, it’s not always about predicting things accurately. But lately, I’ve been working with my brother. He has a B2B business, very traditional. Everything goes based on a handshake, and everything needs 10 people involved. I’ve been working with him to start to think about, Okay, how do we add in data things so that a person doesn’t need to look at inventory levels in order to decide whether we need to order or not? A little bit on the personal side, but we’ve been working together to try and see what we can do to modernise a very traditional industry. The industry is actually fragrance-making. They work with manufacturers from a whole bunch of countries, and they bring in the raw ingredients that are needed for fragrances. Then he’s based out of New Jersey, and that’s one of the hubs for all of these fragrances that get made. For example, celebrity fragrances and stuff like that. So very traditional industry. We’ve been doing some work to try and modernise some of the operational side of things.
VM: That’s beautiful. I just learned something new. I didn’t know that New Jersey was a hub for fragrances.
ST: Paris and New Jersey. Paris and New Jersey, two places in the world.
VM: What? I love that. I love that. Never knew that. Okay, now going to our topic of discussion, Sam. And as you are an expert in data and in the fintech industry as well, we’d love to know more about the impact AI and machine learning are having in the fintech and payment space. So I guess my first question would be, how do you see AI and machine learning currently being used within the payments and fintech space? Are there some cool users that you’ve seen during your time at Wealthsimple?
ST: Absolutely. AI right now went through a few…The term AI went through a few different iterations as to what it meant. Before ChatGPT, AI was always something that was 10 years away. You always spoke about machine learning or algorithms. Right now, everything’s AI just because a lot of eyes and focus are on it. If we think about Fintech, they were the early pioneers in or the finance space or the payment space. They were early pioneers in machine learning models to detect fraud because that was the biggest pain point in the space, and a lot of time and energy went into it. Something else people don’t think about often is just the simple fact of forecasting demand or having a good forecast for cash flow or anything like that is you’re using algorithms and machines to derive that. That’s where it all started or one of the early use cases and that still continues. So any fintech or a finance company you speak to, they are still doing fraud and they’re still doing forecasting models. And to be honest with you, I see that continuing moving forward because that’s just so fundamental to it. More recently, we’re seeing things like support also get an AI angle to it. We’ve seen Klaviyo, most recently working with OpenAI to develop a support chatbot. They also have actions baked into it where some things like resolutions, et cetera, et cetera, for disputes were being automated, and they had a lot of success in being able to automate that. That’s been an interesting angle with support. Of course, we see education being another piece just because a lot of education is blogs or PDFs or written content. And like, GenAI is fantastic to consume that and to also make it personalised for whoever might be interested in talking about or getting educated in that space. And then lastly, we’ve also seen really cool use cases to activate structured data leveraging GenAI and like, Float comes to mind here. What they’ve done is you have an account and you’re using it to buy or sell things. That is all very structured data. “I paid, I got gas, home insurance, car insurance, et cetera, et cetera.” Each of those transaction, of course, has a lot of metadata associated with it. Time of day, day of week, location, what category does this transaction belong to? If you also have your paychecks being directly deposited to that account, now you have cash flow analysis that you can do. Traditionally, activating this data meant someone somewhere needed to write a query and then create a dashboard on top of it and then hope the client is able to extract insight from that dashboard. But now with GenAI, you can give this structured data as context and give people the freedom to ask the query that they are interested in and get the insight right away. That type of leveraging Gen AI to bridge the gap from having a very long winded pipeline to activate data to being able to give people the flexibility and freedom to do as they please is really interesting and enticing. That’s, I think, been one of the cool things coming out in the space.
VM: Lovely. I think you’re right. Absolutely. I think the first time that I thought or if I had to give AI a face, I think ChatGPT was that first face. Because when you go into the more in-depth stuff of fraud prevention, and as you mentioned, all these different use cases, you don’t really have a face for it. I guess ChatGPT firstly became face of AI, and for the general public or perhaps just me.
ST: Sorry. On that point, that has benefits and also that comes with some things to overcome. The benefits are if you now give someone a text box to type in prompt, if they use ChatGPT, now suddenly your product can function without a lot of handholding on how to use the product. Because a lot of people are familiar how to navigate a prompt. The thing to overcome is a chat-based or prompt and chat-based interface is not the only way to use large language models. How do you overcome the inertia where everything needs to be a chat-based prompt to building a product that is leveraging the same underlying technology, but in a different distribution package? I think that’s something that we’re seeing the industry start to navigate to interesting places.
VM: Interesting. Would it be in a way that it understands already what is required? What its role would be instead of a chat and response angle?
ST: I think as a technology, we can assume large language models are just going to get better and better. If we put, are we going to get AGI in the next 5 years, 10 years, question aside, because I think that’s quite nuanced. What we get to is now since 2017, when the “Attention Is All You Need” paper came out and the transformer architecture that now got us to ChatGPT. That was, 2017 to now was a lot of time with incremental improvements. Suddenly with ChatGPT, we had an aha moment where we had a explosion, almost like a Cambrian explosion of ideas of what we could do with a large language model. The underlying tech was able to realise, at least partly, some of those aspirations that we all had for a long time. We can assume the underlying tech is going to get better. But to say that we have exhausted the world of ideas where this tech can be applied, then I think we’re still in the naive phase of figuring all of those out. Because if you look in the industry, there’s a huge diversity of ideas and executions, but they’re not that many. And you would think a tech as transformative as a large language model, there’d be more. So what we do internally is go in with the assumption that all of the places where large language models or AI or Gen AI needs to be applied, go in with the assumption that we haven’t exhausted the list of ideas. And the way we generate new ideas is to give everyone in the company access to this incredible tool in as generic form as possible and see what they do. Then what we realised is… So internally, of course, we’re a fintech,- so we have to build things in a privacy and security lens. We did that with a tool that we call the LLM Gateway. It’s actually open source. We have a blog post about it. It’s a GitHub repo. People can go use it. Should they or implement it in their own world should they choose to do so. But essentially what it did is give everyone access to a very powerful large language model that they can use in any way that they want. And then we noticed what they’re using it for. And part of it was for code, completion, generation, debugging. So then we partner up with GitHub Copilot to say, okay, there’s a specialised tool for this. You don’t need to go out of your IDE where you’re coding every day to go to another tool to use it. Here’s a very specialised tool. Let’s go and see what is the efficiency gains there. Then we also noticed there were customer support people that were looking at the tickets or the issues that our clients were coming to us with and trying to analyse them to say, Hey, what are the types of problems people are coming to us with? We took that use case and we created a large language model-based classification system that on a daily basis looks at every single ticket that we get from our client, whether it’s chat, voice, or email, and categorises it to these 115 categories that we have worked with the business to establish. Now suddenly you have a classification system that no one’s entering any prompts into it but still leveraging Gen AI to beable to classify every single ticket. What this gives us, so just as an aside on the ticket, now suddenly you have a feedback loop where you release a product and immediately within the day, many times it’s just hours, you’re getting feedback from our clients about what they like about it, what they don’t like about it. Now we have an LLM essentially allowing us to quantify how our clients are using it and what needs to get better. That’s fantastic. We have this tool that is going out and automating client issues. Then we took a look at a further use case where people were wanting to analyse data that they were getting it. We said, Okay, let’s build. Internally, we call it a CSV Wizard, where it’s like you bring in a CSV, and then you ask a very targeted question, like how many times did blah happen? Now we have a tool that does that, and then so on and so forth. We started with a very general playground that is safe and privacy-aware. Then we went to each one of the use cases and we said, How do we now build a tool that does this really well? I don’t think we’re done. I think it’ll be many years before we’re done. Then in some cases, we found vendors that we feel very comfortable partnering with. And we said, Okay, what you’re bringing to the table is exactly what we wanted to build, and your approach to this is very much aligned with how we’re thinking about things. Let’s bring you on so that we can accelerate how we deploy these tools to our teams. So you asked the question, what are the ideas coming up? I think the ideas are like we have a huge shelf of ideas, but I also don’t think that is all the ideas that we’re going to come up with. As we play around with this tool and learn more things and understand its capacity more, then I think a lot more crazier ideas, I hope are going to come out of it.
VM: No, that’s lovely. You’re basically making operations more efficient by doing this. You gave two very good examples of how you’re using it in the customer service space as well as internally as well, where a team member doesn’t necessarily have to exit their space they’re in to use something to get a code that they can then use to do their task. It’s right in front of them. I love the efficiency part of it. But what challenges were associated with putting this together? Because I’m assuming it’s a bit of a test to even get this out there. So what was the challenges, firstly, with the customer service space and then with the internal space as well?
ST: Yeah. With this level of tooling coming in, and when I say this level, the revolutionary change that ChatGPT and a tool like that is bringing on, that’s a large change. And anytime you have incremental change management is a pain, right? And it needs to be done carefully. And now when we’re introducing or starting to introduce tools where a lot of things are changing in how we work, in what is required from people to use the tools efficiently,v then there is a lot of change management that needs to be done. One thing that I like to talk about in this space is people, especially in the customer support org, the world is very deterministic. A ticket comes in, you identify what the issue is, and then you look through your knowledgebase on if this is the issue, how do you solve it? And you follow the step and you close out the ticket. Now suddenly, we go from that world to a world that is probabilistic because all AI and ML models are probabilistic by nature. To go from a world that is deterministic and use the same tools and apply them in a probabilistic world, that’s going to be, in some cases, disastrous. Because a 51% fraudulent case is very different from where you have an exact label saying, is this account fraudulent or not? Or is this transaction fraudulent or not? How do you then partner with the organisation that you’re deploying these tools into and work with them step by step to make sure that not only is the technology delivered and it works amazingly, but then the people that are going to be working with it are comfortable, feel empowered, feel like their voices are heard and they feel like they’ve been given the training and the tools to use the technology in the right way. So that, I think, is the biggest challenge that we’re going to face and that we are acutely aware of at Wealthsimple as well, particularly in the customer service or customer support use cases. So what we’ve done is the use cases that we’ve come up with, they’re actually bottoms up. So we went to the customer support agents and we shadowed them. Then we debrief with them to say, Hey, these are the things that you’re doing. We have a potential tool that is able to help you out. This is how you use it. This is all the areas in which you need to second guess it. These are the things that it does really well. Now, what else do you think is prime for introducing in their workflow, leveraging this technology? Then when you work with the people, that’s when you get the buy-in and you get the uptake of the tool, and the tool can really deliver value. In all of these cases, what we’re talking about is the tool as a copilot to help the human. If we are in the business of helping humans, then we can help more of our clients, and we can help the humans really focus on the things that only humans can do, which is human to human communication and add the human element to any of our interactions.
VM: Lovely. I love the copilot aspect that you brought to it. I also, I don’t know why I imagine Sam sitting with the customer service team with a notepad just writing, this is what you could have a copilot on. I love it. Sam, I’d love to ask, once you did that and once you deployed some of these tools to the customer service team, what results did you see in terms of how it was helping or how it was impacting talking with clients? So what feedback or results did you see?
ST: Yeah. So if I can take the example of us classifying or categorising every single ticket that comes in. Now, suddenly you have a quantitative view of what your entire funnel of tickets look like. Which topic of tickets take the longest time to resolve? Which topic of tickets have the most reopening rates? Which topic of tickets are being funnelled to the team that is least staffed to be able to handle it? When you add a quantitative layer to all of these things, then your operating model changes, how your staff changes, what training you provide changes, what are the tools that you bring online to support these teams changes. That is one area where as a net effect of having this data does is it gives you more ideas to help the people that are on the ground. We’ve seen efficiencies in our operating model as a result of this. Then what happens is the product team that are building products, they have access to the same data. Then they are looking to see how can we leverage this data to then prioritise our roadmap coming up. Because people are really asking about this feature that we don’t have. People are really asking because this onboarding flow is very confusing. This is a fundamental mental change in how we operate as a company by having this feedback mechanism, all powered by this LLM tool that we had built.
VM: I love that. And then you can do so much with it, right? And another aspect that you shared was how you could implement certain tools to help, for example, coders. So how has the Wealthsimple team welcomed – obviously, product and customer service makes sense because they’re one is obviously customer-facing, and then the results or the feedback that you get, the product team can use it to come up with some solution that clients are seeking currently. But for people such as perhaps marketing or coders, how has AI been received by them?
ST: So just taking a further step back, though, some of you look at the tools that we built and if we aggregate it, a half of the company uses it on a monthly basis, and a third of the company uses it on a weekly basis. We have very benchmarking ourselves against other peers, that’s a fairly high level of penetration in terms of strictly LLM tooling, and that is strictly internal. So I’ll share with you one use case that maybe comes from a team that is not traditionally thought of as needing tools like this. So we have an advisory team and we have a BDR team, and both of them talk to our clients on a regular basis. And these teams, they need information about the clients that they’re speaking to, and they need information about the company and our products at a very detailed level. Now, Wealthsimple, we are shipping products constantly, to have a up to date information about when a particular product is going to ship in the future, what are the current offers going on right now. What are the accounts that someone has currently access to? Et cetera. That’s a lot of cognitive burden for these agents or these advisors to hold on to, as well as the BDR agents to be aware of. What we built are actually two tools. One tool takes all of the structured data for a client and is able to summarise it in a very easy to use manner so that a person before they jump on a call with a client, they do research. This becomes a tool that they can do to do a very thorough research. We built that through interviewing many of the advisors and being like, as you’re doing research for the clients, what are the types of things that you are interested in? And we went, found that data, and then summarised it in a very easy to consume manner while being very thorough and accurate. And on the other end, we built a chatbot that takes in all of our marketing material, all of the articles that have gone out as being announcements from Wealthsimple it has access to a Google Drive that has all of decks from our marketing folks and our product marketing folks, and people are able to search that and only that information to then unlock things like, Hey, when are USD accounts going out? Okay, let me quickly look that up, where instead of following up with the clients, they’re able to, in context, look up this information and feed it back to the clients while they’re on a call with them. You don’t think about advisors or BDR folks leveraging these types of tools,but by sitting with them and understanding their needs and also very well understanding what the technology can unlock, we’re able to build these very specific tools that’s able to accelerate the service that they’re providing while improving the quality in many of these cases.
VM: I love that. You just laid out so many, avast amount of benefits that AI can potentially bring to so many different teams. On the advisory side, I couldn’t really think of that. That’s an interesting one. I like that one. But I guess my last question, Sam, would be, you mentioned this earlier in the conversation where you said that it’s a constant improving process when it comes to AI and what you could do with it. But what do you see in the next few years? How will AI evolve and how do you see it playing a role in the fintech industry as even new things emerge, such as open banking and conversations about the real-time rail? So how do you see AI in the next five years when it comes to the fintech industry?
ST: Yeah. One thing, especially in the fintech space, there’s a lot that regulations have to speed up in order to allow for some of these innovations. I mentioned the LLM gateway that we have internally. We actually have a team of security people, privacy people, legal people, regulatory people, and data scientists all working together to make that product go live. That just means it’s a lot of complexity. That complexity is still to be disseminated in rules and laws and best practises. In the next five years, what I would hope is that there is an acceleration in known best practises on how we can deploy some of these technology-level things that are coming out. Now, as a net effect of that, as you’ve seen with our story, we started on a ladder. We start with understanding how to use these tools internally, and then we build tools so that internal folks are using them to support clients. And then the next rung on the ladder is building tools so that clients use products built with this technology directly. And what I would hope is in the next five years, not only are there more ideas in each of these rungs, but they are way more customer-facing products that are entirely being powered by large language models. Now, that could be generative AI or AI in general. That could be a few different things. It could be strictly an education thing. It could be strictly a research. You’ve seen a lot of products in the industry now where the public statements that any public company makes, their financials that they put out, all of them are being scoured and then research via large language models. I think we’re going to see a proliferation and a lot more usage of those types of technology being client-facing. What we saw with the Float example of taking your own data and then unlocking it so that you can make decisions on your own, I foresee a lot more of those things where you’re not opinionated about the decisions that needs to be made, but you’re unlocking the ability to consume large amounts of data in order to make decisions. So I think that’s really fascinating, and I’m curious to see how this space evolves. And selfishly, we’re also thinking about working and building tools that will enable a lot of these cool things to be a reality.
VM: And I have no doubt that…You’re going to absolutely do that whenever you get all the data with you. But Sam, I really want to thank you for joining us. And this is one of the most, I guess you can agree with me when I say this, that AI, I think 2024 and '23, it’s been the hottest topic that everyone has spoken about. I think this conversation needs to happen where you understand within an industry that deals with technology and finance, how are these tools being utilised and what are they utilised being for? Everyone has an idea of, Hey, it’s used for fraud prevention. But from an advisory standpoint, customer standpoint, so much to learn. I really want to thank you. For me, this was the 101 on what AI can do in fintech. So thank you so much for this. And yeah, thank you so much. Amazing.
ST: Awesome.Thank you for having me.
VM I want to thank Sam Talasila, the head of LLMs at Wealthsimple for joining the study and explaining how artificial intelligence is impacting the fintech industry. If you have any questions for us or Sam, please do comment them down below. Don’t forget to like, share, and subscribe to our YouTube channel. Thank you so much for tuning into the Simplify Payments podcast presented by Paramount Commerce. I’m your host, Varad Mehta, and I’ll see you soon.